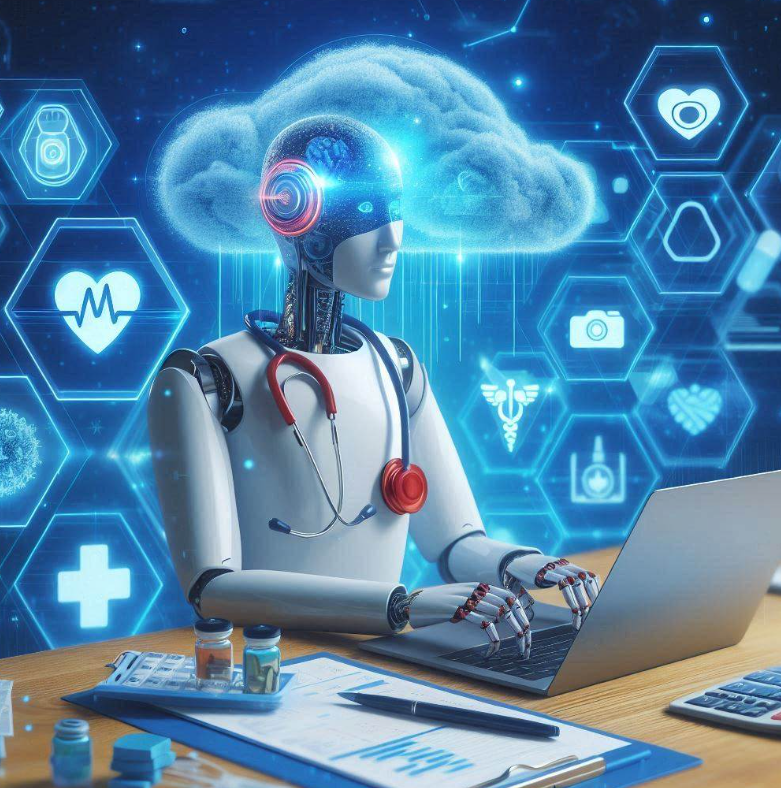
Artificial Intelligence (AI) is revolutionizing various sectors, and healthcare is no exception. The integration of AI into medical coding and billing processes is not only enhancing efficiency but also significantly improving accuracy and compliance. This article delves into how AI is reshaping the healthcare industry, particularly focusing on medical coding and billing.
The Role of AI in Healthcare
AI’s impact on healthcare is profound, offering solutions that streamline operations, enhance patient care, and reduce costs. AI technologies, such as machine learning and natural language processing (NLP), are being utilized to analyze vast amounts of medical data, predict patient outcomes, and assist in clinical decision-making. These advancements are paving the way for a more efficient and effective healthcare system.
Transforming Medical Coding and Billing
Medical coding and billing are critical components of the healthcare revenue cycle. Traditionally, these processes have been labor-intensive and prone to human error. However, AI is changing the landscape by automating many of these tasks, leading to significant improvements in accuracy and efficiency.
- Automation of Repetitive Tasks: AI-powered systems can automate repetitive tasks such as claim submission and denial management. This not only speeds up the billing process but also reduces the workload on human staff, allowing them to focus on more complex tasks.
- Enhanced Accuracy and Compliance: AI algorithms can analyze clinical documentation and assign appropriate billing codes with high accuracy. This reduces the risk of errors that can lead to claim denials and compliance issues. AI systems continuously learn and adapt to new coding guidelines, ensuring ongoing compliance with industry standards.
- Improved Revenue Cycle Management: By automating various aspects of the billing process, AI helps healthcare providers manage their revenue cycles more effectively. AI can identify patterns and trends in billing data, enabling predictive analytics that optimize billing workflows and reduce claim denials.
- Fraud Detection and Prevention: AI plays a crucial role in detecting and preventing fraudulent activities in medical billing. By analyzing large datasets, AI can identify anomalies and patterns indicative of fraud, helping to safeguard the integrity of the billing process.
- Real-Time Auditing and Feedback: AI-driven solutions provide real-time auditing and feedback, ensuring that medical codes are accurate and compliant with regulations. This reduces the time and effort required for manual audits and enhances the overall quality of the billing process.
Challenges in Implementing AI for Medical Coding
While AI offers numerous benefits, its implementation in medical coding and billing is not without challenges. Healthcare providers must navigate several obstacles to successfully integrate AI into their systems:
- Data Privacy and Security: AI systems require access to vast amounts of sensitive health data, raising concerns about data privacy and security. Ensuring that patient information is protected and complies with regulations like HIPAA is a significant challenge.
- Integration with Existing Systems: Integrating AI solutions with existing Electronic Health Record (EHR) systems can be complex. Compatibility issues and the need for seamless data exchange between systems can hinder the implementation process.
- Lack of Skilled Personnel: There is a shortage of skilled professionals who can develop, implement, and maintain AI systems. Training existing staff to work with AI technologies is essential but can be time-consuming and costly.
- Algorithmic Bias and Fairness: AI algorithms can sometimes exhibit biases based on the data they are trained on. Ensuring that AI systems are fair and do not perpetuate existing biases in healthcare is a critical challenge.
- High Initial Costs: The initial investment required for AI implementation can be substantial. This includes costs for software, hardware, training, and ongoing maintenance. Smaller healthcare providers may find these costs prohibitive.
- Regulatory and Compliance Issues: Navigating the regulatory landscape for AI in healthcare is complex. Ensuring that AI systems comply with all relevant regulations and standards is essential but can be challenging.
- Resistance to Change: Healthcare providers may face resistance from staff who are accustomed to traditional coding methods. Overcoming this resistance and fostering a culture that embraces technological innovation is crucial for successful AI implementation.
Mitigating Algorithmic Bias in AI Coding Systems
Mitigating algorithmic bias in AI coding systems is crucial to ensure fairness and accuracy in healthcare. Here are some strategies healthcare providers can adopt:
- Data Preprocessing:
- Bias Detection: Analyze and clean the data to identify and remove biases, ensuring the training data is representative of diverse patient populations.
- Balancing Data: Use techniques like oversampling underrepresented groups or undersampling overrepresented ones to create a balanced dataset.
- Algorithm Modifications:
- Fairness Constraints: Incorporate fairness constraints into the AI algorithms to ensure they do not favor any particular group.
- Regular Updates: Continuously update and retrain AI models with new data to ensure they adapt to changing demographics and medical practices.
- Post-Processing:
- Outcome Analysis: Analyze the outcomes of AI predictions to check for biased patterns and make necessary adjustments.
- Feedback Loops: Implement feedback mechanisms where healthcare professionals can report discrepancies or biases, allowing for continuous improvement.
- Transparency and Explainability:
- Explainable AI: Use AI models that provide clear explanations for their decisions to understand how decisions are made and identify potential biases.
- Documentation: Maintain thorough documentation of the AI system’s development, including data sources, preprocessing steps, and algorithm choices.
- Diverse Development Teams:
- Inclusive Teams: Ensure that development teams are diverse, bringing in different perspectives and reducing the risk of embedding biases into algorithms.
- Bias Training: Provide training for developers and healthcare professionals on recognizing and mitigating biases in AI systems.
- Regular Auditing and Monitoring:
- Continuous Monitoring: Regularly audit AI systems to detect and address biases, monitoring performance across different demographic groups.
- Third-Party Audits: Engage independent third parties to audit AI systems for biases and provide recommendations for improvement.
Conclusion
AI is transforming the healthcare industry by revolutionizing medical coding and billing processes. By automating repetitive tasks, enhancing accuracy, improving revenue cycle management, detecting fraud, and providing real-time auditing, AI is paving the way for a more efficient and effective healthcare system. Despite the challenges, the potential benefits of AI in medical coding make it a worthwhile endeavor for many healthcare providers. As AI continues to evolve, its applications in healthcare will expand, offering even more sophisticated solutions for medical coding and billing.
Feel free to share your thoughts or ask any questions in the comments below!